Let me start with a quick update on something Substack related. Throughout the last month I attended an invitation-only weekly forum hosted by Substack called Substack Go where writers were brought together to help encourage each other. For me writing on Substack is generally a solitary activity that happens mostly on Saturday morning. That effort is followed by a Sunday morning of editing and expansion. Finally the rest of the week is spent tinkering to get to a final post that goes out on Friday. A lot of the content being presented during Substack Go is about how to craft and write a newsletter which is interesting to hear about. This particular series has reached 58 weeks and the weekly rhythm of writing and publishing seems to be working. Even my new podcast variation of this series has been working.
The two topics that I have spent the most time writing about within the machine learning space are strategy (ROI/KPIs/budgeting) and training efforts like bringing somebody or a team up to speed. Training is wholesale an element that should be a part of any organizational ML strategy. This post certainly could start by harkening back to Week 4 that appeared on February 19, 2021. During that week I tackled the topic, “Have an ML strategy… revisited.” Contained within that analysis were two questions targeting “What exactly is an ML strategy?” and “Do you even need an ML strategy?” The answer to that pivotal question is still of course an organization should have a machine learning strategy. Beginning with the end in mind and having that direction tied to budget level KPI is really a minimum standard at this point.
Within this analysis the question really is if teaching or training machine learning skills is the right path to take. Inherent within that analysis has to be a question about the reason for wanting to learn the machine learning skills or acquire that specific knowledge. Bringing team members up to speed is certainly a reason to champion the training process for introducing machine learning skills. On the other side of that consideration is a scenario where learning about the foundations and building up knowledge inspired the need to pick up machine learning skills. In that case the collecting of knowledge simply compels more collecting. Continuing this general line of thought about training; I am going to break up the next two sections into concentrated coverage of individual enrichment followed by coverage of team building.
Individual enrichment as a reason for advancing machine learning skills covers a significant, but not overwhelming number of people that comprise this use case universe. Students and lifelong learners alike see the world of machine learning and either have a fear of missing out or are wondering what the hype is all about. The hype in the marketplace is all about a new technology that can be applied to business use cases. People look at potential and are willing to make a leap to what it could possibly mean for them or what it could possibly do for the organization. Within the machine learning space going from model to production is a journey. Even the process of keeping a model well tuned and running in production is a consideration that means that the journey never really ends.
Team building or more to the point the individual practitioners within teams or solo efforts that advance machine learning within organization certainly comprise the overwhelming bulk of the people contained in this use case universe. People at all sorts of different skill levels are working and learning within the machine learning space. Very few of them have the knowledge, skills, and abilities to create a machine learning system like TensorFlow or PyTorch. The preponderance of work in the machine learning space is done on the shoulders of giants based on using frameworks and systems that were already built. However, we are starting to see a lot of the newest innovations coming out of organizations like Hugging Face and EleutherAI. The developers with the skills to produce those foundational platforms and tooling that people are going to be using in the machine learning space will continue to be a tier above the much larger pool of people using the products. That asymmetrical dynamic is unlikely to change.
To end this post, I thought I would provide you with a short update on my audio editing strategy. Within the Audacity software I’m using three different effects to clean up my podcast audio. First, a noise reduction effect which I have used for a long time in the product is kicked off and executed. The noise gate effect would probably catch it all, but I still run it first. Second, a loudness normalization effect is run which is key for any podcast as it makes sure nothing shocking in terms of a volume spike happens to the listener. Third, I have started using the built in noise gate effect function to trim out background noises and any breaths that might remain. Between those three effects in Audacity my podcast audio is being edited for your listening pleasure.
Links and thoughts:
From Machine Learning Street Talk, “#063 - Prof. YOSHUA BENGIO - GFlowNets, Consciousness & Causality”
“Valve's Making Everyone Else Look Bad - WAN Show February 18, 2022”
“AI Show Live | Ep 52 | Analyze unstructured docs and more with Azure Form Recognizer”
Top 5 Tweets of the week:


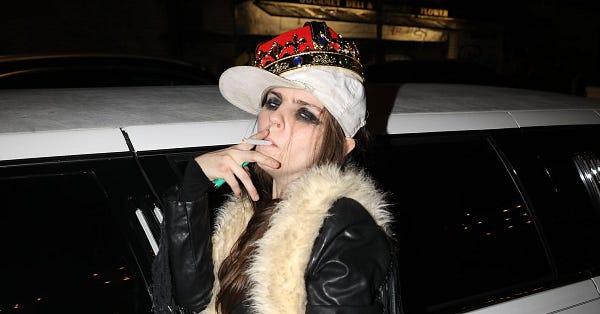

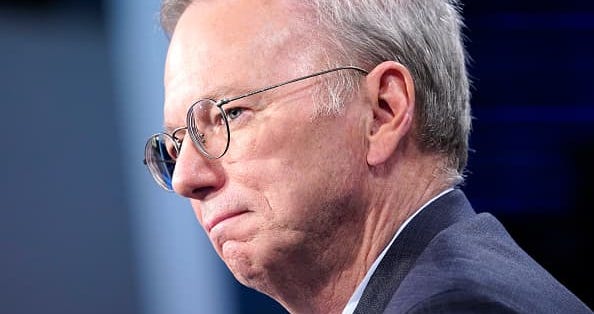

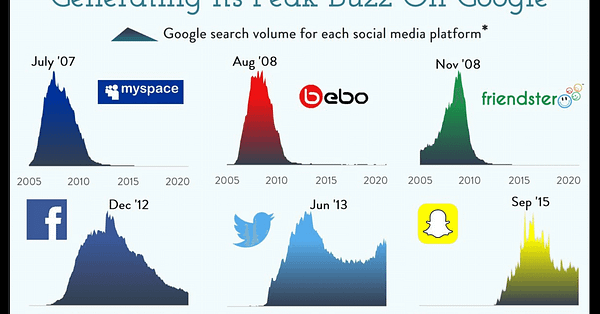
Footnotes: None.
What’s next for The Lindahl Letter?
Week 59: Multimodal machine learning revisited
Week 60: General artificial intelligence
Week 61: AI network platforms
Week 62: Touching the singularity
Week 63: Sentiment and consensus analysis
I’ll try to keep the what’s next list forward looking with at least five weeks of posts in planning or review. If you enjoyed reading this content, then please take a moment and share it with a friend.
Share this post