Prompt engineering and machine learning
During the very long car ride back to Kansas City from Denver for Thanksgiving this year, the whole family got to listen to an audiobook called “The Age of AI: And Our Human Future” by Daniel Huttenlocher, Eric Schmidt, and Henry Kissinger (2021). Outside of an oddly lengthy aside about the history of philosophical considerations going back to Plato that snuck into a chapter. You will know it when you get to it in the book. The book itself was a pretty good read and provided solid examples of where artificial intelligence currently stands. They were super quick to point out limitations at the point where the book was written.
One of the things about working within the space of modern machine learning is the very real necessity of prompt engineering and having people that understand it. Is this just a very fancy method of leading the witness? That could very well be the case. The cornucopia of large language models that are being released seemly monthly now are all pretty much interacted with based on prompt engineering.[1] The other day I spent some time looking at the Megatron-Turing large language model and trying to consider how much bigger a model could get before the langue it was modeling would be exhausted.[2] To me it was sort of a modernity adjacent version of the Borges' Map fable. To exhaustively map everything would seemingly be an effort to create a mirror of modernity. At 530 billion parameters it is an exceedingly large generative language model. These models are super interesting and you might recall that paper from a ton of Stanford University professors, “On the Opportunities and Risks of Foundation Models.”[3] This paragraph took a bit to get to the point of this missive that these types of models are here to stay and they require a special skill set called prompt engineering.
To engage in prompt engineering people are going to face challenges getting the expected outputs.[4] You work with the model by giving it prompts or writing a predefined prompt package to make it create outputs from the model. During the course of building my own bot using the GTP-2 model I learned really quick that even with millions of words of my own writing corpus it required the right type of prompt to get output from the model that matched my writing style.[5] That is what makes prompt engineering so interesting in terms of this supremely large language models. People spend so much effort compiling, training, and packaging these generative models (foundation models) that needing a specialized skill set to work with them is an interesting situation to have occurred.
Links and thoughts:
“AI Show Live - Episode 41 - Best of AI Show Holiday Edition!”
“PC Gaming is Officially the BEST! - WAN Show November 26, 2021”
Top 6 Tweets of the week:

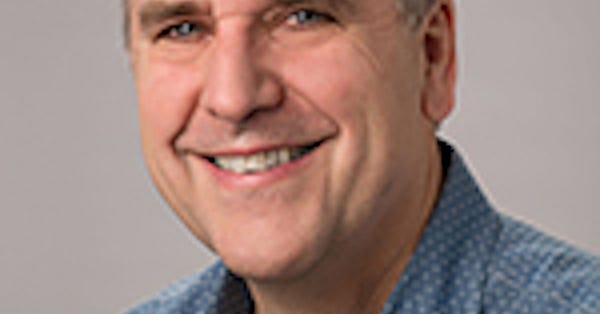

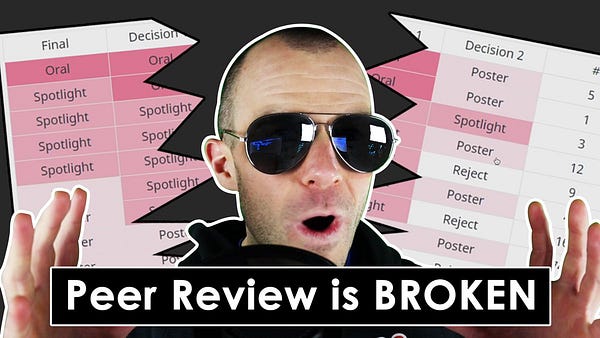

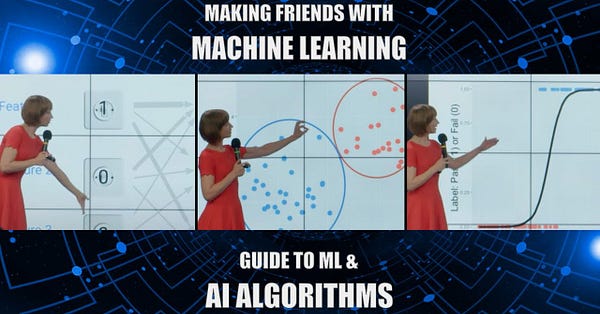

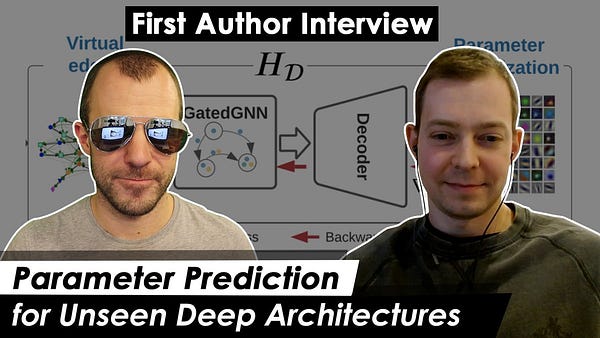

Footnotes:
[1] https://blog.andrewcantino.com/blog/2021/04/21/prompt-engineering-tips-and-tricks/
[3] https://fsi.stanford.edu/publication/opportunities-and-risks-foundation-models
[4] http://ai.stanford.edu/blog/in-context-learning/
What’s next for The Lindahl Letter?
Week 46: Machine learning and deep learning
Week 47: Anomaly detection and machine learning
Week 48: Machine learning applications revisited
Week 49: Machine learning assets
Week 50: Is machine learning the new oil?
Week 51: What is scientific machine learning?
Week 52: That one with a machine learning post
I’ll try to keep the what’s next list forward looking with at least five weeks of posts in planning or review. If you enjoyed reading this content, then please take a moment and share it with a friend.