Plan to grow based on successful ROI
Whoa... the number of machine learning and AI newsletters has grown out in the wild internet based pages of Substack and Revue (Twitter owns that one). I was trying to figure out the differences between Substack and Review by searching and reading Twitter feedback from users. More prominent uses are on Substack it seems to work ok. At the moment, I’m going to stay with Substack for a few more weeks and see how it goes. More people seem to be excited about and using Substack as a platform than are using Revue within my online bubble.
I’m going to try to weave in some of my Tweets into these newsletters that directly link back out to things that really caught my interest each week. Here is the first example of that:

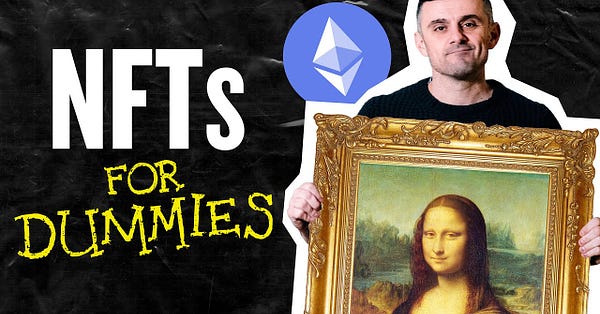
Be careful searching for machine learning growth strategies in a search engine. That one is going to follow you around for a while. You are going to get all sorts of advertisements back.[1] During my journey down that search path, I read an estimate that the machine learning market is going to end up around 8.81 billion dollars in 2022.[2] That estimate seemed interesting enough at first glance. That might have been due to the context of a recent article I read about Google building out something called “Model Search'' which is essentially an open source platform for finding machine learning models.[3] That really caught my attention. A ton of use cases exist and models have been trained for a lot of them. Being able to get going without the cost of training a model yourself could kickstart your effort. I had an idea about it that I’ll share in a couple weeks. It could turn into a project at some point.
Executing a plan can be very rewarding. When that plan is tied to budget level key performance indicators with solid return on investment the rewards are even quantifiable. You have to plan to grow based on successful return on investment. That happens when spending is tied to budget items that are geared toward successful outcomes. Part of that is about knowing your key performance indicators and managing the process along the way and the other part is about having the fiscal discipline to build return on investment models and execute that strategy. Yeah planned growth is harder than it sounds. You will find that really building out a layered plan to get things done involves operationalizing your strategy vision and executing it in a planful way. Some of that is low hanging fruit for the organization and other parts are more complex algorithmic use cases.[4]
Getting a really solid list of the use cases where growth could be driving is a challenge worth attempting. That is more than just engaging in cost avoidance or reducing ongoing operational expenses it is about finding the places where growth is really possible. That is really a core part of understanding the business and ultimately should be very closely tied to how the budget is defined. A really solid strategic plan would tie the right investments to the right places going forward. That sounds really simple as a sentence, but it is really hard to do in practice. The places where growth was possible could change and shift within the year. Some efforts to move forward will be more successful than others. That is why you have to have the right key performance indicators to drive and guide evidence of progress or to shine the light on areas that need help from the leadership team to stay on track.
I really enjoyed this post about GPT-3 https://investinginai.substack.com/
You can catch Rob’s latest podcast here: https://www.buzzsprout.com/1697077/8085144-investing-in-ai-episode-2-heath-terry-from-goldman-sachs
I watched over an hour of this video from Machine Learning Street talk
That lead me to want to read and subscribe to this https://marksaroufim.substack.com/p/machine-learning-the-great-stagnation
Footnotes:
[1] Check out this fun little article from Forbes https://www.forbes.com/sites/forbesinsights/2020/05/08/realizing-the-growth-potential-of-ai/?sh=1f25069033f3
[2] https://www.marketsandmarkets.com/Market-Reports/machine-learning-market-263397704.html
[3] https://www.marktechpost.com/2021/02/28/google-ai-introduces-model-search-an-open-source-platform-for-finding-optimal-machine-learning-ml-models/ you can see it here on GitHub https://github.com/google/model_search
Top 5 Tweets of the week:

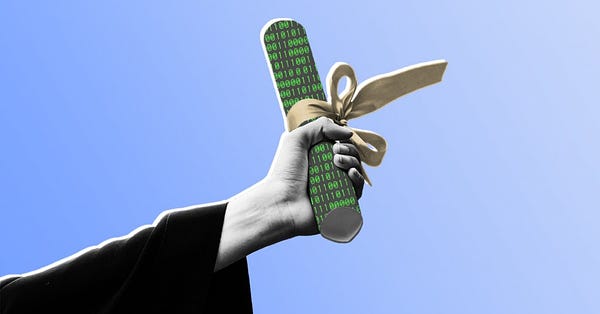

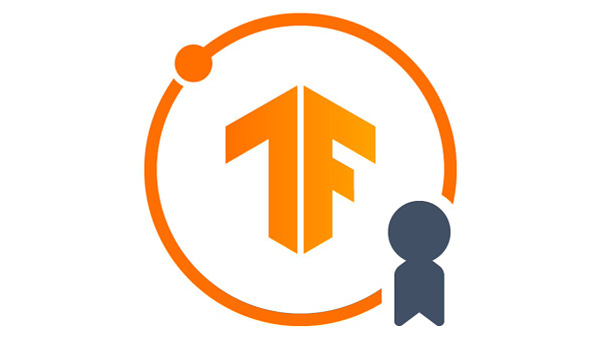

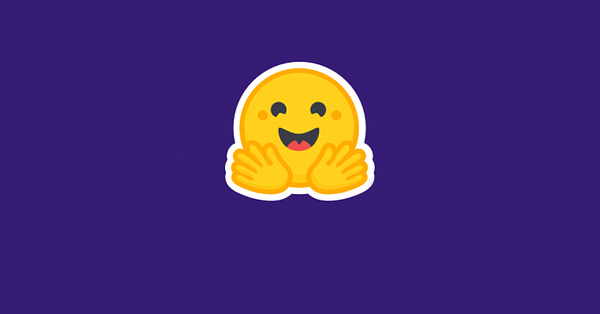

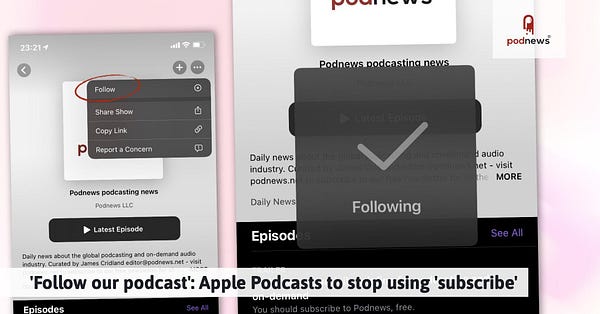

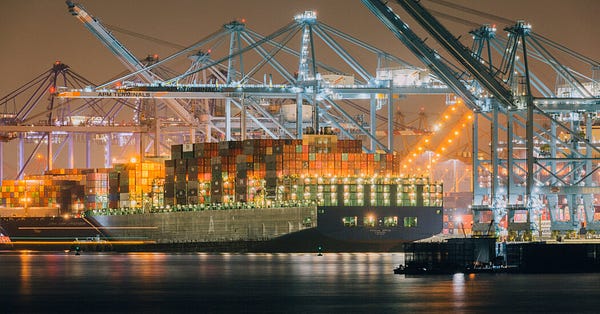
What’s next for The Lindahl Letter?
Week 8: Is the ML we need everywhere now?
Week 9: Valuing ML use cases based on scale
Week 10: Model extensibility for few shot GPT-2
Week 11: What is ML scale? The where and the when of ML usage
Week 12: Confounding within multiple ML model deployments
I’ll try to keep the what’s next list forward looking with at least five weeks of posts in planning or review. If you enjoyed reading this content, then please take a moment and share it with a friend.