You might remember back to Week 75 when I tried to explain the nature of overcrowding within the machine learning space and what exactly that is doing to engineering colleges. Back in 2020, Igor Susmelj wrote a piece in Towards Data Science, “How to Keep up With the Latest Research and Trends in ML” [1]. Within that paper, Igor raised awareness of a chart from Jeffrey Dean that showed machine learning arXiv papers versus Moore’s law growth rate [2]. A quick Google Scholar search of that paper will show that it is cited by 72 papers at the moment [3]. I had hoped that a batch of papers would help me find more academic work about overcrowding and machine learning, but that did not really turn out to be the case. I have been trying to figure out the right words to describe intellectual overcrowding and machine learning, but for the most part I have not been able to figure out the secret decoder ring settings to locate a bunch of papers on the subject.
I noticed a chart on page 10 of a paper called, “An Overview on Applications of Machine Learning in Petroleum Engineering,” which showed the number of publications with AI/machine learning within the SPE OnePetro digital library [4]. Just like the chart from Jeffrey Dean, it showed a clear takeoff point after 2010, where the terms just skyrocket like a hockey stick. That is probably a fairly consistent trend, and things will shake out over time to a key set of academic articles that get referenced a lot and a core set of topics that are covered within the AI/machine learning academic community. At the moment, however, we are at the peak of the inflection point, where intellectually everybody rushed to be first in the pool and kept on swimming.
With a rush of academic focus in the area of machine learning, we will see both a great deal of progress and a potentially calamitous fallout from intellectual overcrowding. Only so much progress and ultimately only so many faculty positions are going to exist within engineering programs. I do think we will see a pretty significant oversupply in the number of people seeking those faculty positions at the more prestigious set of academic institutions. Right now it is a lot more lucrative for people who are able to get jobs within industry to do that and to enjoy some pretty solid compensation. That is probably the element that has made the overcrowding less problematic during the rise of machine learning implementations. People being able to work in the private section and people working within academic spaces have been able to find work. You can find a lot of articles about brain drain within academic institutions related to both AI and machine learning. Some of those pieces make some interesting arguments. A lot of private organizations have run labs that are doing things of a more academic nature than perhaps applied use case development.
Links and thoughts:
Top 4 Tweets of the week:

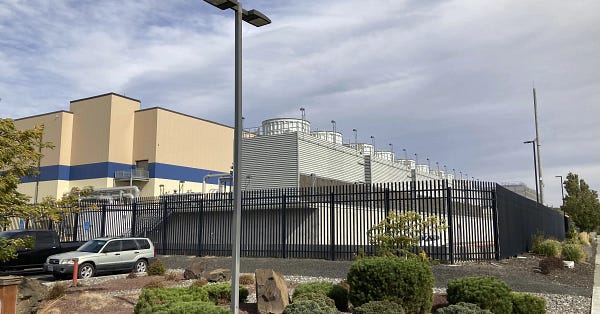

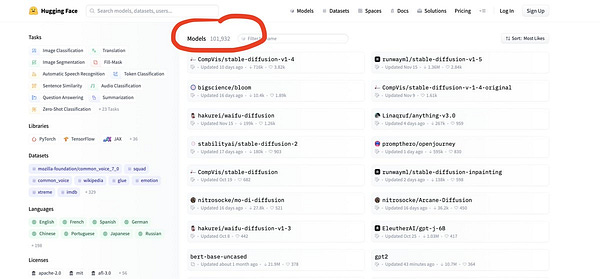

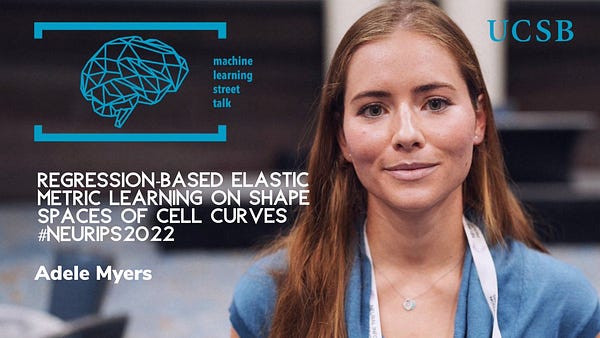
Footnotes:
[1] https://towardsdatascience.com/how-to-keep-up-with-the-latest-research-and-trends-in-ml-a45a356b1001
[2] https://arxiv.org/ftp/arxiv/papers/1911/1911.05289.pdf
[3] https://scholar.google.com/scholar?cites=3848695121612936760&as_sdt=4005&sciodt=0,6&hl=en
What’s next for The Lindahl Letter?
Week 101: Back to the ROI for ML
Week 102: ML pracademics
Week 103: Rethinking the future of ML
Week 104: That 2nd year of posting recap
I’ll try to keep the what’s next list forward looking with at least five weeks of posts in planning or review. If you enjoyed this content, then please take a moment and share it with a friend. If you are new to The Lindahl Letter, then please consider subscribing. New editions arrive every Friday. Thank you and enjoy the week ahead.
Share this post