Machine learning feature selection
Well the buzzword of the week very quickly went from #Metaverse to #TimeCrystal and I guess that means science is winning. It was very hard to switch it up from reading about time crystals to writing about machine learning feature selection, but for you, for continuity, and for the planned posting schedule I don’t want to waste a second; here we go!
The more time you spend working in the machine learning field you will get tired of hearing about overfitting. Training to a use case or to a commonality of the data is an interesting thing to consider. Given enough time you can navigate the best possible answer to a given training dataset or to the bias and limitations of the total dataset being split apart for training. Training is a major part of the adventure and any model you work with could become overfit to the training data and it won’t work correctly in production.[1] That is why feature selection and training the model on the right things is critical to success.
What exactly is feature selection? You are going to have to train your machine learning model on some type of data. It would be a very interesting conversation to hear about models that require no data. It would be very interesting indeed. Your data is going to be made up of some things you are going to work with and model around which is going to require you to define the features. Maybe for example you want to predict the price of houses based on location, size, and year constructed. Part of the art of data science is either working with the features to find the right match for your model or sometimes it is easier to use automated methods that work through the possible features that you have in your dataset to locate potential combinations. That might sound super complex, but the idea of feature engineering and the methods behind selecting the right features has its own special place in the field of machine learning
Links and thoughts:
This short video from Leo and team was pretty good “What is a Time Crystal?”
Check out Susan Athey during this Stanford Graduate School of Business talk about “Machine Learning and Economics: An Introduction”
I watched TWIG with Leo, Ant, and Jeff this week with Mike Masnick on “The Evil Ceramicist - Bye bye Google ads, how to fight disinformation, Pixel 6 Pro, YouTube Shorts”
This week during the writing process I did have Linus and Luke on in the background. They are sort of like my Saturday morning cartoons I guess. “I Have MORE to Say About Steam Deck - WAN Show August 6, 2021”
Go check out Yannic Kilcher this week “[ML News] AI-generated patent approved | Germany gets an analog to OpenAI | ML cheats video games”
Top 5 Tweets of the week:





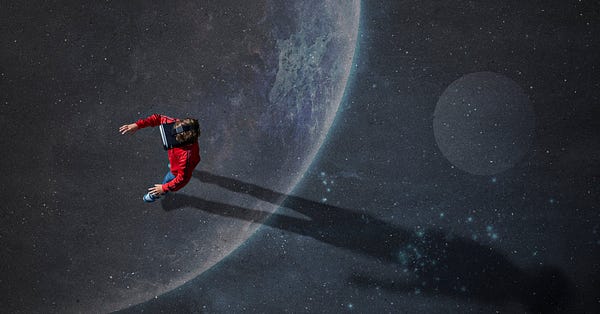

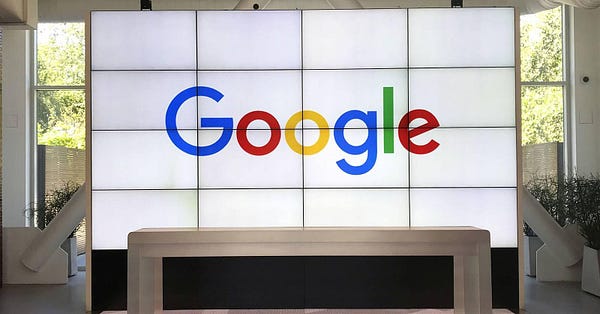
Footnotes:
What’s next for The Lindahl Letter?
Week 30: Integrations and your ML layer
Week 31: Edge ML integrations
Week 32: Federating your ML models
Week 33: Where are AI investments coming from?
Week 34: Where are the main AI Labs? Google Brain, DeepMind, OpenAI
I’ll try to keep the what’s next list forward looking with at least five weeks of posts in planning or review. If you enjoyed reading this content, then please take a moment and share it with a friend.