Machine learning assets
Right now you are reading the last of my words written for 2021 (week 49 of The Lindahl Letter) and assembled in this post about machine learning assets. This week was a bit of a game changer as I started work on a new paper/presentation for my 2022 talks. The title of that presentation will be, “The next 5 years of ML in the healthcare space.” Right now I’m thinking that the presentation will be broken into 3 parts: 1) a healthcare space mapping, 2) plotting the next 5 years, and 3) where will ML be in that 5 year mapping of healthcare. That bit of news now provided let’s get back to the heart of this post about machine learning assets.
Most of my searching for machine learning assets scholarly related research pointed me in the direction of how you elect to manage your resources or equipment that run machine learning jobs or other efforts. The research I stumbled into was very tactical in nature and not very strategic. I had thought a few more scholarly articles about the management of machine learning as an intellectual property asset would be more prominent. Instead what bubbled up was a lot of content about interfaces to control and launch your machine learning efforts from a browser. This is one way to look at machine learning assets as a literal view on a screen, but not really the finance heavy definition of asset I was looking to learn about this week. I was curious about the economic value of machine learning assets a company or individual might generate.
Every single time you work on a machine learning project you start to develop assets. These are either going to be considered valuable or it's possible they will not yield any real depreciable asset. This topic is like a flashback to an article in the Harvard Business Review from 2019.[1] That article takes a turn into how machine learning can help manage financial assets vs. a more traditional question about how the machine learning tools themselves should be treated as assets on the books of the business. It is easy to look at the expenditure of funds to build a piece of software as something that can be deprecated. You can also consider that a model built for machine learning might have value outside the walls (increasingly virtual walls) of the company. Most of the marketplaces where you can buy and sell models are not super expensive places to do business. It seems like the proliferation of models has lowered the value.[2] Maybe the commodification of models and the very expensive cost of building large language (foundational) models has influenced the value. Some people are even using machine learning to mine intellectual property data which was interesting to read about.[3] I also ended up reading about this digital watermarking technique for neural network models that was really surprising.[4] I’m not sure how they would ever see the watermark in practice.
You could take this in a different direction and consider the nature of the competition between open source elements and highly proprietary ones. A lot of the main elements of the machine learning workflows are either open source or highly proprietary locked down to APIs. Some things like the GPT-3 model are purely proprietary and API driven. A lot of the more expensive models are served up via API and that is very different from the open source roots of TensorFlow, Scikit-learn, PyTorch, and Keras have been shared via scholarly efforts or open source repositories. The open source nature of things might explain why most organizations do not have large swatches of intellectual property in the machine learning space.
Links and thoughts:
“[ML News] AI learns to search the Internet | Drawings come to life | New ML journal launches”
“How to bring electricity with you using a power station (buying guide)”
This could be my next mouse it looks amazing “TUF GAMING M4 AIR MOUSE| ASUS”
“RadioShack Returns - WAN Show December 24, 2021”
Top 6 Tweets of the week:



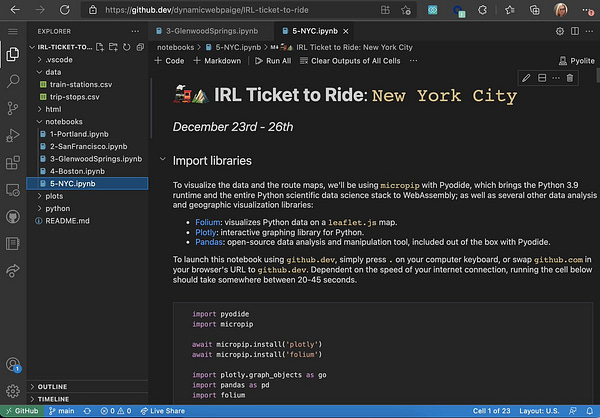


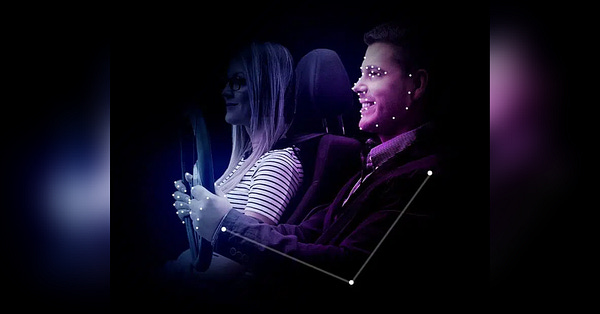

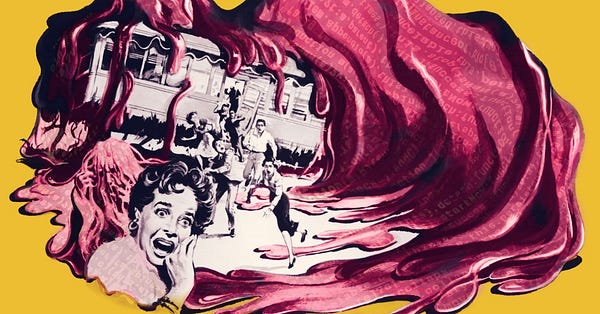
Footnotes:
[1] https://hbr.org/2019/12/what-machine-learning-will-mean-for-asset-managers
[2] https://aws.amazon.com/marketplace/solutions/machine-learning
[3] https://www.sciencedirect.com/science/article/pii/S0172219018300103
[4] https://dl.acm.org/doi/abs/10.1145/3196494.3196550
What’s next for The Lindahl Letter?
Week 50: Is machine learning the new oil?
Week 51: What is scientific machine learning?
Week 52: That one with a machine learning post
Week 53: Machine learning interview questions
Week 54: What is a Chief AI Officer (CAIO)?
I’ll try to keep the what’s next list forward looking with at least five weeks of posts in planning or review. If you enjoyed reading this content, then please take a moment and share it with a friend.