Machine learning and deep learning
Our friends over at IBM are eager to answer questions about the differences between general artificial intelligence, artificial intelligence, deep learning, and machine learning.[1] I’m listening to Bruce Springsteen’s song Darkness on the Edge of Town while working on this post this morning. You get questions of a digital divide, ethics, and equality starting to be generated as you evaluate all the different aspects of the above mentioned technologies. My treatment of the terms here is really about functionality and not the potential consequences of that functionality. I’ll take a look at that during a future Substack post, but not explicitly during this turn of the wheel.[2] You can rest assured that Timnit Gebru (formerly of Google) is now hard at work on this topic with a new think tank the Distributed Artificial Intelligence Research Institute (DAIR).[3] We took an important veer to the philosophical side on the introduction here to the topics in question. A lot of complexity exists within both the technology and the applications of the technology in question. Both sides of those concerns have to be considered; just because you can do a thing does not mean that you should. While the logic in that last sentence is easy for you to parse and understand as a reader it does not parse in a model with the same ethical frame of reference you bring naturally to the process.
You might have snuck ahead to that link from the IBM blog and you already know that general artificial intelligence is a desired future state (or at least much anticipated future state) where an instance of artificial intelligence could handle multiple scenarios like a person does. Right now most of the work in artificial intelligence is defined to a specific problem and use case thus making it a special case and not a general case. Both machine learning and deep learning are going to end up being a highly focused effort to do a specific thing or to learn about a specific set of content. Our friends over at IBM would be quick to explain in length that deep learning is a subset of machine learning.[4] Generally speaking that is an easy assertion to make, but it might not celebrate deep learning in the way it deserves as a truly interesting method of discovery. You can do a ton of different types of machine learning based on algorithmic approaches and at some point you will run into a problem where you have something like a ton of images you want to work against a neural network with layers to deeply learn features and elements. When you veer into deep learning you are unlocking some capabilities in the machine learning space that are at the very least very interesting.
Links and thoughts:
“The Government is SUING NVIDIA? - WAN Show December 3, 2021”
“The Essential Tool Kit For PC Builders”
“The Best Laptops - 2021 Picks!”
Top 5 Tweets of the week:

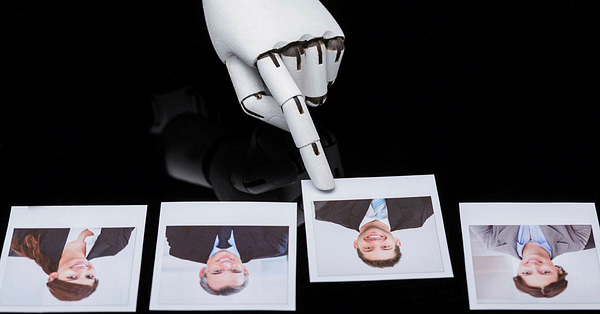



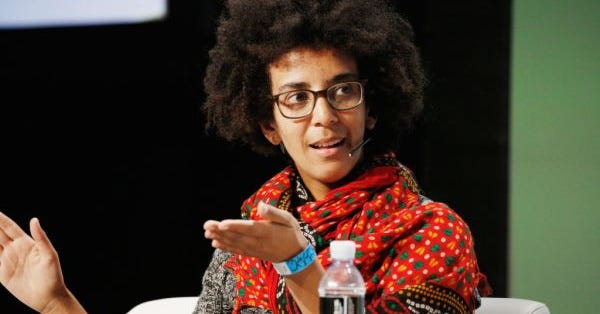

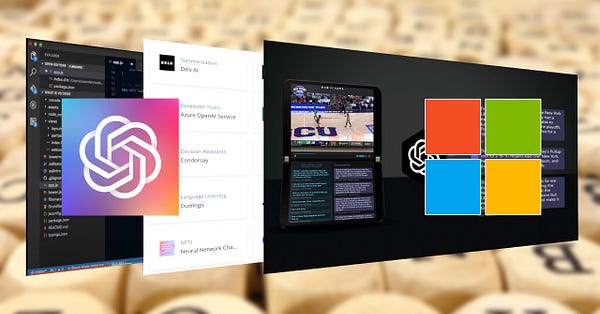
Footnotes:
[1] https://www.ibm.com/cloud/blog/ai-vs-machine-learning-vs-deep-learning-vs-neural-networks
[2] Right now weeks 64 and 65 are queued up for a posts on ethics in machine learning and a question about machine learning and the digital divide
[3] https://www.washingtonpost.com/technology/2021/12/02/timnit-gebru-dair/
[4] https://www.ibm.com/cloud/learn/deep-learning
What’s next for The Lindahl Letter?
Week 47: Anomaly detection and machine learning
Week 48: Machine learning applications revisited
Week 49: Machine learning assets
Week 50: Is machine learning the new oil?
Week 51: What is scientific machine learning?
Week 52: That one with a machine learning post
I’ll try to keep the what’s next list forward looking with at least five weeks of posts in planning or review. If you enjoyed reading this content, then please take a moment and share it with a friend.