Is the ML we need everywhere now?
Just as you read that title that the ML we need is everywhere now. Some of you just looked at your Apple watch. You don’t have to look very far to see some application of machine learning in your daily life. People have been making the argument that machine learning is everywhere for years.[1] Now if machine learning has become that obvious in your day to day existence you can only imagine how deeply it is starting to get ingrained in the business world.[2] Pretty much every vendor, every application, and every underlying technology is investing in some combination of machine learning and artificial intelligence.[3] This creates some really interesting situations where you have machine learning models working in unanticipated compounding and confounding ways. Yeah, I’m really proud of that last sentence. I’m invested in the combination of talking about how models can be compounding and confounding. That is probably just a loquacious way of saying the models either have working results or conflicts develop.
We have reached a point where businesses may not be aware of all the different machine learning elements running in the organization. They could even be running at cross purposes. A chatbot could be trying to answer simple questions and close out the conversation as quickly as possible with very little friction for the customer. Another element of machine learning could be running to evaluate what options, opportunities, or suggestions the organization has for the customer and that entire line of conversation might never be engaged. You have to remember that targeted optimization algorithms could be at odds with an engagement strategy. You could also have different parts or programs or different elements of a complex workflow working at very different purposes and outcomes along the way. That type of confounded interdependencies will create havoc for your overall machine learning strategy. It is probably evidence of either a failed machine learning strategy or no real strategy at all.
Check out this video linked below from the Microsoft team explaining how to run Notebooks out of VS Code
I watched this 8 minute episode of Cord Cutting Weekly and thought about Netflix and the future of login drama
I watched over 60 minutes of this video from Machine Learning Street Talk this week that includes a chat with Christoph Molnar
Check out this podcast Land of the Giants “The Moonshot Factory” https://megaphone.link/VMP1924119223
Top 5 Tweets of the week:



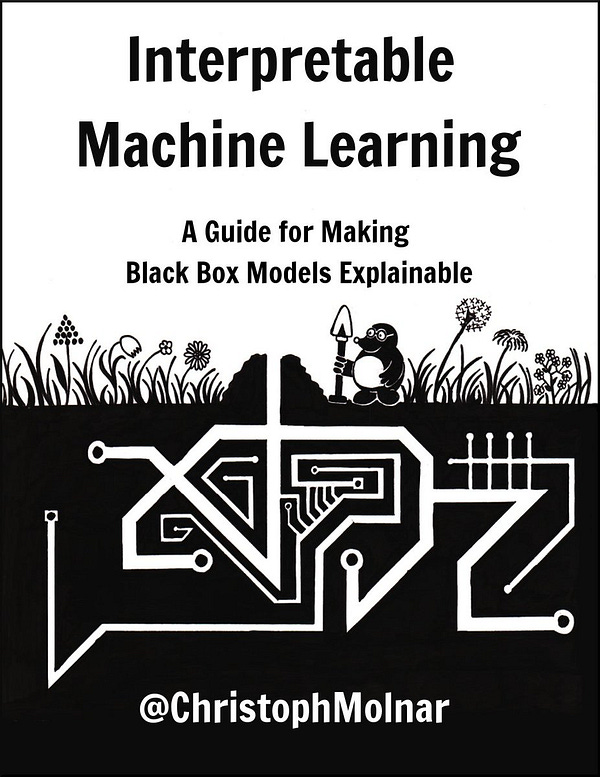

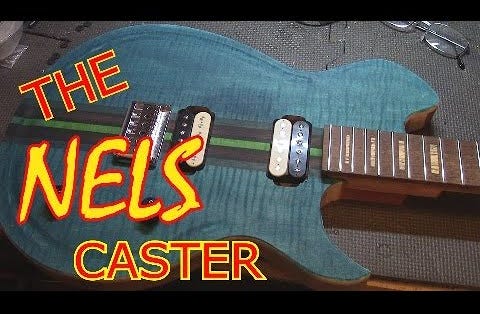



MLOps Spotlight #1
Company: Databricks
Product: Managed MLflow
Github: https://github.com/mlflow/mlflow
Offering: Full machine learning life cycle management (which sounds a lot like MLOps spelled out in my detail) including experiment tracking, model development, model deployment, and security features. Two offerings exist including open source MLflow and a service of managed MLflow on Databricks that includes more features.
Footnotes:
[1] From 2017 https://www.datanami.com/2017/07/03/machine-learning-everywhere-preparing-future/
[3] https://www.nytimes.com/2021/02/23/technology/ai-innovation-privacy-seniors-education.html
What’s next for The Lindahl Letter?
Week 9: Valuing ML use cases based on scale
Week 10: Model extensibility for few shot GPT-2
Week 11: What is ML scale? The where and the when of ML usage
Week 12: Confounding within multiple ML model deployments
Week 13: Building out your ML Ops
I’ll try to keep the what’s next list forward looking with at least five weeks of posts in planning or review. If you enjoyed reading this content, then please take a moment and share it with a friend.