Within the broader generative AI space, the part I tend to focus on is related to the written word. Right now, all of the visual generation parts of generative AI in terms of images and videos are wholesale living in the public mind [1]. Creative people are generating thumbnails and playing with all sorts of plausible image generation technology. A few teams are rapidly working on how to make video from that same type of generative AI and that is going to be interesting. We are probably going to see generative AI shows that people create very soon. I have previously written (Week 78) that I think all public trust in imagines is going to erode and that we are going to hit a zero-trust wall when it comes to being able to believe what we see [2]. This missive will be about the future of where large language models are going and a bit of a reflection on what has happened in the last couple of years.
Back on October 26, 2021, the folks over on Hugging Face shared out a post called, “Large Language Models: A New Moore's Law? [3]. This post starts out with a very familiar graphic of the models in terms of billions of parameters over time. This is a relatively recent phenomenon with a start during 2018 and massive acceleration after 2020. You may well have heard about the Megatron-Turing natural language generation model (MT-NLG) [4][5][6]. You can imagine that people were thinking they should make larger models. After all what is better than a billion-parameter model? It obviously has to be a trillion-parameter model. I would argue that the reality of having unique parameters within that large of a search space is probably something that is being disregarded at this point, but that has not stopped the march for more and more parameters. You might be thinking that nobody has really done that type of effort in practice.
The M6 model happens to be 10 trillion parameters [7]. Yeah, a 10 trillion parameter model exists. That is mind bogglingly large.
One of the longest papers with the most authors was published by a lot of people from Stanford was “On the opportunities and risks of foundation models” [8]. You probably could have guessed that I was going to throw the title of that paper into Google Scholar to see what other people were adding with that citation to the scholarly world aka the academy [9]. That query offered up about 565 results to consider.
Bommasani, R., Hudson, D. A., Adeli, E., Altman, R., Arora, S., von Arx, S., ... & Liang, P. (2021). On the opportunities and risks of foundation models. arXiv preprint arXiv:2108.07258. https://arxiv.org/pdf/2108.07258.pdf
This link will take you right to the 412 papers that Google Scholar believes have a direct citation to that massive 212 page paper [10].
One of the real concerns when that paper got published was that it covered so much ground and had so many coauthors that it would very quickly become an anchor citation that was heavily cited. Some people were worried it would just become a stock or default citation for people in literature reviews. I’m pretty sure that the number of scholars that came together on that work will pretty much guarantee that it gets cited a ton going forward. The other element that will help with that is that the paper is highly readable for people wanting to learn and understand large language models. Together those two elements of it being useful to read and known by a large number of scholars from the start pretty much guarantee that people will hear about it for years to come.
Links and thoughts:
“[ML News] Multiplayer Stable Diffusion | OpenAI needs more funding | Text-to-Video models incoming”
“We've Made Some Big Mistakes - WAN Show November 18, 2022”
Top 5 Tweets of the week:

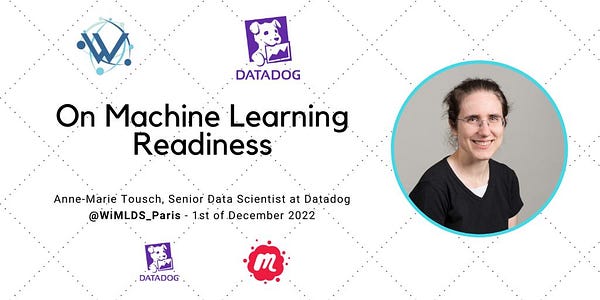


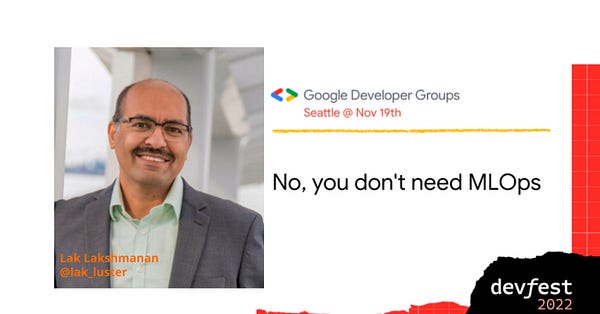
Footnotes:
[1] https://venturebeat.com/ai/how-2022-became-the-year-of-generative-ai/
[2]
[3] https://huggingface.co/blog/large-language-models
[4] https://developer.nvidia.com/megatron-turing-natural-language-generation
[5] https://arxiv.org/abs/1909.08053
[7] https://towardsdatascience.com/meet-m6-10-trillion-parameters-at-1-gpt-3s-energy-cost-997092cbe5e8
[8] https://arxiv.org/pdf/2108.07258.pdf
[10] https://scholar.google.com/scholar?cites=9595110325981705564&as_sdt=4005&sciodt=0,6&hl=en
What’s next for The Lindahl Letter?
Week 97: MIT’s Twist Quantum programming language
Week 98: Deep generative models
Week 99: Overcrowding and ML
Week 100: Back to the ROI for ML
Week 101: Revisiting my MLOps paper
Week 102: ML pracademics
Week 103: Rethinking the future of ML
Week 104: That 2nd year of posting recap
I’ll try to keep the what’s next list forward looking with at least five weeks of posts in planning or review. If you enjoyed this content, then please take a moment and share it with a friend. If you are new to The Lindahl Letter, then please consider subscribing. New editions arrive every Friday. Thank you and enjoy the week ahead.
Share this post