Federating your ML models
Research note: Make sure to go read this 212 page paper, “On the Opportunities and Risks of Foundation Models,” that was published by a huge number of people from Standard University.[1] I’ll be reading it; albeit slowly, this weekend.
Based on the number of citations (1,267) a paper called “Federated Machine Learning: Concept and Applications” by Yang back in 2019 has drawn people to it at least as a starting point.[2] It seems to pop up very quickly within any searches you might start firing up on the subject. You could rewind a little further back in time to Thursday, April 6, 2017 and see a post from the Google Research team that details federated learning.[3] That post by McMahan and Ramage occurred years earlier and might very well lead you to a paper that is cited by about 2,852 researchers called, “Communication-Efficient Learning of Deep Networks from Decentralized Data.”[4] You can start to get a good foundation from those references about federated learning.
The idea of federated learning is pretty simple and it allows you to work on machine learning across several (even many) devices without exchanging the original base local data. You then bring all the learning elements back together which based on the distribution creates the federated part of federated learning. It was and remains a very novel method of building out robust machine learning without data privacy and in some cases data transmission problems. Probably the best understood example of where federated learning is awesome has come from our efforts to build self-driving cars. They generate and abandon huge volumes of data while the sensors are running. It would be nearly impossible for all the Tesla cars to send all that data back for analysis. Instead of trying to manage it that way, federated learning allows distributed efforts without all that backhauling of the data which is really pretty interesting as a method and should be appreciated.
Links and thoughts:
Yannic made this video you might enjoy, “[ML News] Stanford HAI coins Foundation Models & High-profile case of plagiarism uncovered”
During the course of preparing this edition of the newsletter I watched The WAN show with Linus and Luke, “YOU CAN INSTALL WINDOWS 11!! - WAN Show August 27, 2021”
You can go check out The AI Show from Microsoft Developer this week, “AI Show Live - Episode 28 - I wanna 🤘🏽 Rock (Paper, Scissors) with Seth”
I’m still pretty salty about Topps being dropped by MLB

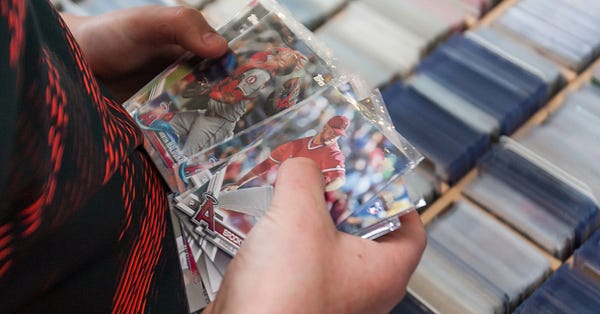
Top 5 Tweets of the week:

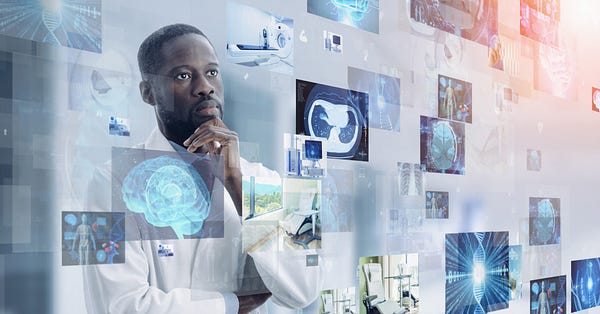


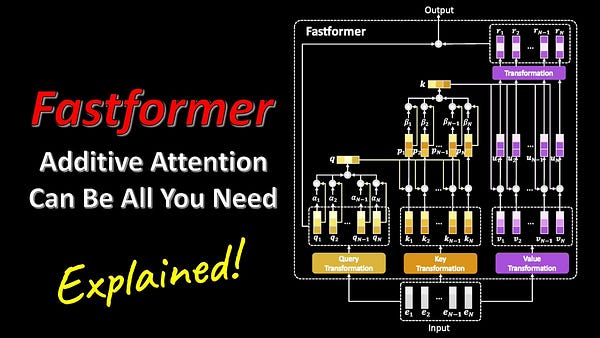

Footnotes:
[1] https://arxiv.org/pdf/2108.07258.pdf
[2] https://arxiv.org/pdf/1902.04885.pdf
[3] https://ai.googleblog.com/2017/04/federated-learning-collaborative.html
[4] https://arxiv.org/abs/1602.05629
What’s next for The Lindahl Letter?
Week 33: Where are AI investments coming from?
Week 34: Where are the main AI Labs? Google Brain, DeepMind, OpenAI
Week 35: Explainability in modern ML
Week 36: AIOps/MLOps: Consumption of AI Services vs. operations
Week 37: Reverse engineering GPT-2 or GPT-3
I’ll try to keep the what’s next list forward looking with at least five weeks of posts in planning or review. If you enjoyed reading this content, then please take a moment and share it with a friend.