Fear of missing out on ML
All that hype leads to a certain degree of buzz. Given enough buzz you almost certainly end up with more hype. That cycle goes up and down within the world of machine learning and certainly within the space of artificial intelligence.[1] We have reached a tipping point where the usability of a lot of machine learning use cases has hit the point where they can go into production without much friction. A lot of more complex use cases face equality complex implementation problems as you go out to operationalize them in practice, but the vast majority of possible ways to start using machine learning are now easy to access. You don’t even have to build out infrastructure or spend a ton of time training models on your data. GCP, Azure, and AWS are all providing easy access to very stable and scalable APIs to fulfil the needs of your machine learning use cases.
All of that buzz and hype mentioned in the first paragraph of this missive has created a fear of missing out on machine learning with the C-Suite of a lot of organizations. Deriving operational efficiency or competitive advantage from machine learning are legitimate things to fear missing out on from machine learning use cases. Right now the possibility of getting return on investment from increasing operational intelligence by catching abnormalities or predicting them is something that can be financially beneficial. Some groups do believe that the amount of hype is excessive.[2] One of the points that Andrew Ng made about this is that people can really showcase the art of possible on a test data set, but getting into the daily hardening of running in production sometimes does not translate to the same efficiency.[3]
Links and thoughts:
Check out this Google Cloud Tech workflow discussion called “Building and training ML models with Vertex” AI
This might just be the future of office work for a ton of people that use Excel and don’t be fooled a lot of people out in the business world use this product “Got Data? Need a front end? Excel add-ins to the rescue! | OD553”
You should probably get to know @datadoghq. This is one of those things that I could see really taking off in the next 2 years based on the rise of multi-cloud implementations “How to Monitor Your AKS Microservices With Datadog | OD484”
Yannic Kilcher has been at it again explaining academic papers with this fine video “Expire-Span: Not All Memories are Created Equal: Learning to Forget by Expiring (Paper Explained)”
Top 7 Tweets of the week:

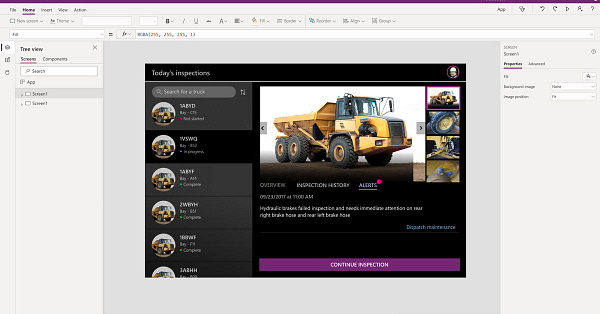

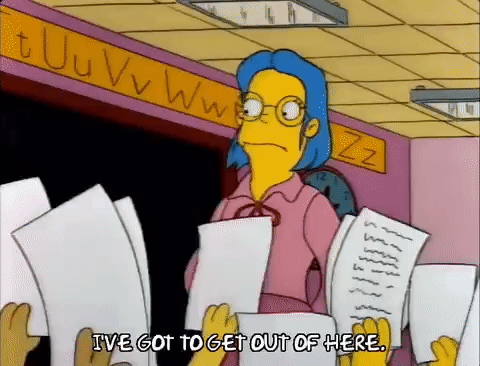

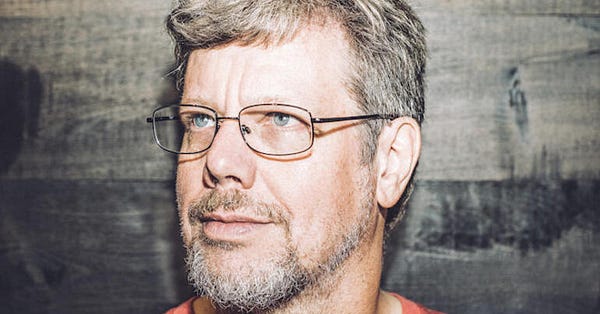

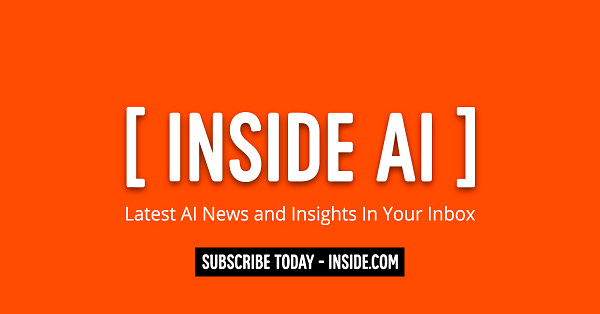


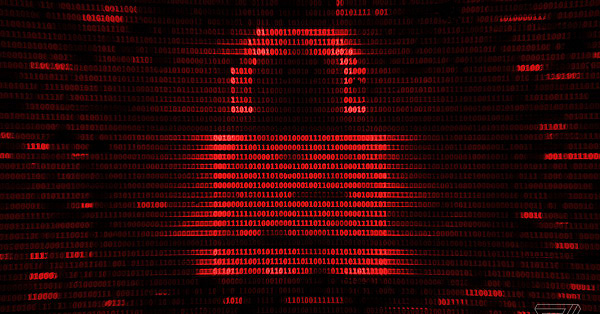

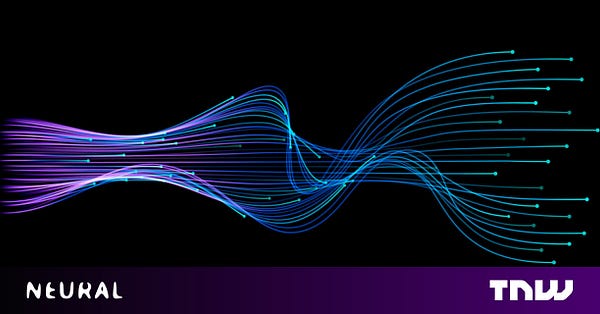
Footnotes:
[1] Here is an example of where even an interesting thing does not stand out in the sea of hype and buzz https://techcrunch.com/2021/05/18/google-cloud-launches-vertex-a-new-managed-machine-learning-platform/
[2] https://www.cioinsight.com/innovation/ai-machine-learning-substance-behind-the-hype/
[3] Andrew Ng talked about this recently https://spectrum.ieee.org/view-from-the-valley/artificial-intelligence/machine-learning/andrew-ng-xrays-the-ai-hype
What’s next for The Lindahl Letter?
Week 20: The big Lindahl Letter recap edition
Week 21: Doing machine learning work
Week 22: Machine learning graphics
Week 23: Fairness and machine learning
Week 24: Evaluating machine learning
I’ll try to keep the what’s next list forward looking with at least five weeks of posts in planning or review. If you enjoyed reading this content, then please take a moment and share it with a friend.