Do most ML projects fail?
You have probably heard that around 85% of machine learning projects fail. That statistic comes from a 2018 Gartner report (press release) that contained the following, “Gartner predicts that through 2022, 85 percent of AI projects will deliver erroneous outcomes due to bias in data, algorithms or the teams responsible for managing them.”[1] For the last 38 weeks (during the course of publishing this Substack) I have been talking about the necessity of having a top level machine learning strategy that prioritizes use cases based on return on investment.[2] You have a ton of pundits and other pontificators giving guidance on how to make your machine learning project successful.[3] To me it is about having a machine learning strategy and executing in a planful way that highlights a definable and repeatable plan to success.
We can fast forward a few years and catch up to current from a newer report Gartner teams shared, “Gartner research shows only 53% of projects make it from artificial intelligence (AI) prototypes to production. CIOs and IT leaders find it hard to scale AI projects because they lack the tools to create and manage a production-grade AI pipeline.”[4] I guess that means the trajectory of success has improved in the last few years, but the prediction from the Gartner team that most (more than half) of ML projects will fail is consistent. This is one of the reasons that I spend so much of my time and speaking appearances talking about open source MLOps efforts. That is where I see the most people working together to overcome the obstacles described above.
Q: What do you do to help prevent your ML projects from failing?
A: Use the patterns that are known to work. Full stop.
A lot of machine learning use cases have been demonstrated to be effective. Start out by targeting in and deploying similar use cases. That is the best method of getting into the machine learning ecosystem and probably getting a solid return on investment from your ML strategy. Doing something that is definable and repeatable from an external API that has been proven durable and dependable is always easier than trying to do something unique within your organization. Some of those use cases could be really rewarding, but they are going to be hard to drive toward success. To that end, people who are just looking to access a machine learning API from AWS, Azure, or GCP are going to find a much easier path to use case success. All of the work is happening to train, implement, and continuously improve the machine learning models by the provider. You also get the benefit of having many many users pressure testing the API vs. being siloed into a single service use case.
Links and thoughts:
This video from Google Cloud Tech’s #VMEndToEnd was pretty good this week, “How to save money with VMs”
This video from Google Cloud Tech’s #ArchitectingCloudSolutions was also descent this week, “How to architect a no-code ML platform on Google Cloud”
From Microsoft Developer on YouTube, “UNITED STATES
AI Show Live - Episode 34 Introduction to Deep Learning”
During the course of editing and extending this Substack missive I did listen to the WAN show with Linus and Luke, “I Have MORE to Say About Steam Deck - WAN Show October 8, 2021”
Top 5 Tweets of the week:

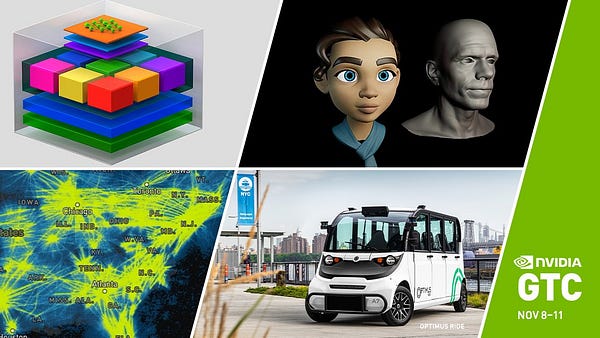

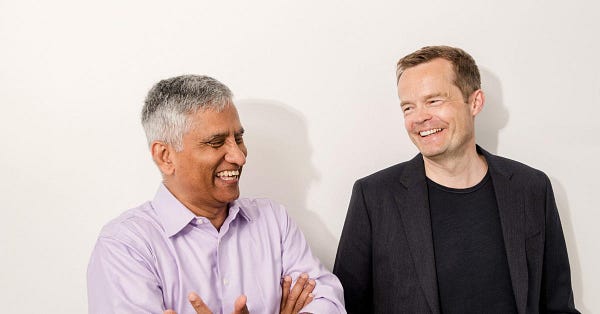

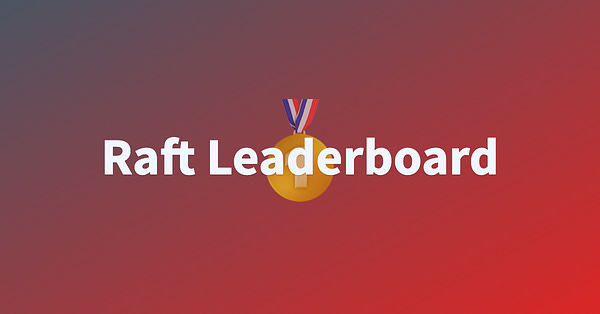

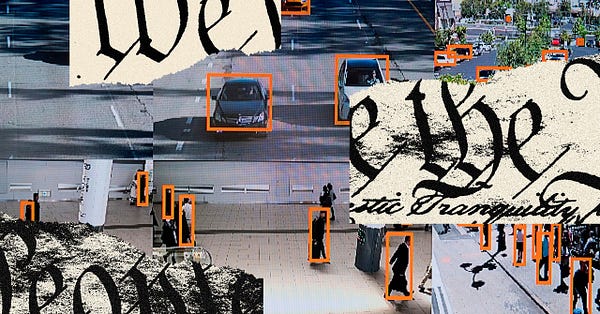
Footnotes:
[2]
and pretty much every talk I have given in the last 3 years
[3] https://www.kdnuggets.com/2021/02/why-machine-learning-projects-fail.html or maybe this one https://towardsdatascience.com/why-production-machine-learning-fails-and-how-to-fix-it-b59616184604
What’s next for The Lindahl Letter?
Week 39: Machine learning security
Week 40: Applied machine learning skills
Week 41: Machine learning and the metaverse
Week 42: Time crystals and machine learning
Week 43: Practical machine learning
I’ll try to keep the what’s next list forward looking with at least five weeks of posts in planning or review. If you enjoyed reading this content, then please take a moment and share it with a friend.