Confounding within multiple ML model deployments
This topic deserves a lot more attention than it got before. Confounding variables can really mess with expected relationships and expected outcomes.[1] A baseball game can be broken down into a definable and repeatable workflow. You could use machine learning models to predict the next most likely pitch a batter will face at the plate.[2] At the same time a model might be engaged from the pitcher's perspective to determine the best possible pitch and location to defeat the batter based on history and other variables. Both of those models can operate without any confounding effects. Both models could be inherently true within the moment. However, the next best action might change for both models based on a series of events within an adversarial model. Introducing an adversarial element to the model could very well be of confounding nature compared to the original intent. A lot of things could happen in this example, but they all follow the general workflow that occurs during a baseball game. A large part of the process is definable and repeatable based on the rules both teams have collectively agreed to and umpires enforce. Model interference is an interesting question to entertain. Depending on the workflow in question you could have a bunch of different machine learning algorithms occurring in a series.
Links and thoughts:
I shared a link to all the Ai4 videos from the conference this week on Twitter

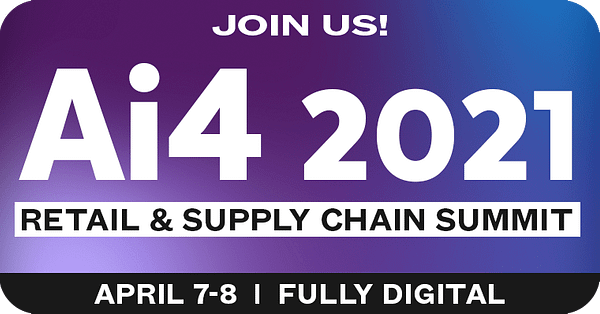
You can get directly to the video of my talk via this link to YouTube
This week I did spent a few minutes (30 or so) listening to Linus and Luke talk during the WAN show about computer hardware. During the pandemic my interest in computer hardware has been rekindled.
This also seemed like a pretty great video from GCP “Migrating WebSphere applications”
You can check out Rob May talking to Kevin Roose during the “Investing in AI” podcast this week

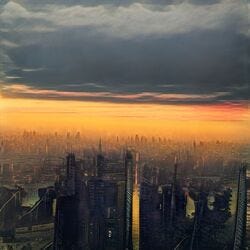
Top 5 Tweets of the week:

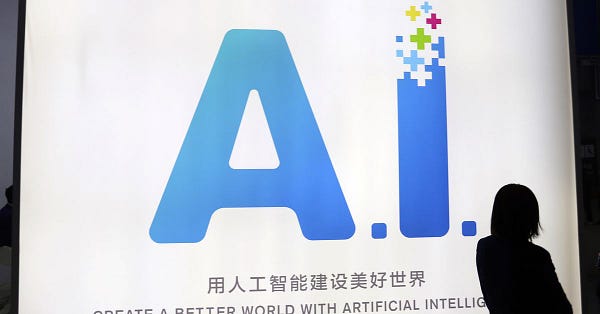

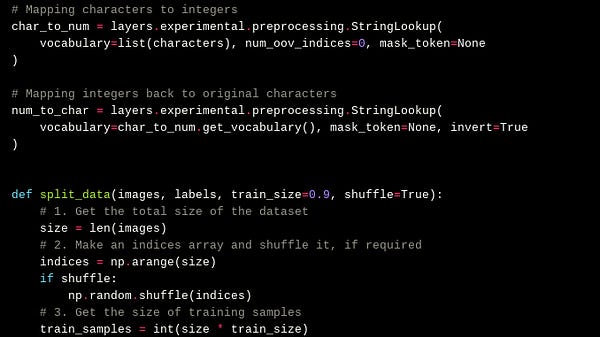



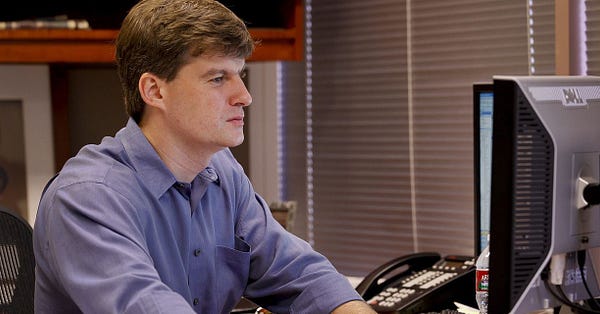

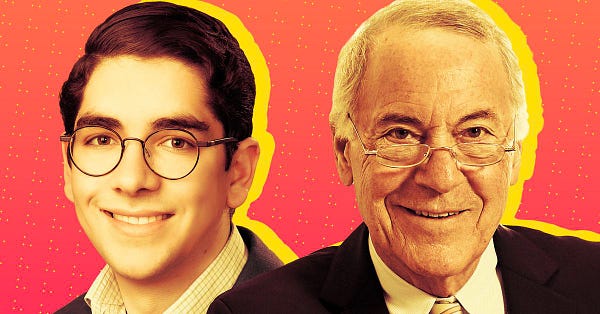
Footnotes:
[1] Check out this sweet video from a few years ago that explains confounding in detail generally as a statistical topic
[2] Check out https://towardsdatascience.com/predicting-the-next-pitch-using-artificial-neural-networks-fc464383f53d or maybe for fun check out this https://medium.com/@matt42kirby/predicting-baseball-pitches-33f906cbbdee
What’s next for The Lindahl Letter?
Week 13: Building out your ML Ops
Week 14: My Ai4 Healthcare NYC 2019 talk revisited
Week 15: What are people really doing with machine learning?
Week 16: Ongoing ML cloud costs
Week 17: Figuring out ML readiness
I’ll try to keep the what’s next list forward looking with at least five weeks of posts in planning or review. If you enjoyed reading this content, then please take a moment and share it with a friend.