Anomaly detection and machine learning
Examples abound from how people are using machine learning to detect anomalies going back 20 years.[1] Let’s take this week's consideration of anomaly detection in two general ways. First, the most interesting example to me of anomaly detection in terms of human life comes from the applied medical research space. Advances in medical imaging have allowed the possibility of using machine learning to detect anomalies in scans in ways that either enhance the process of detection or that help direct doctors to take a closer look at specific images. Second, anomaly detection is all over the security space. Given systems where data is generally consistent in that space any abnormality could be pattern matched to help prove an outlier is an anomaly. Between those two spaces of medicine and security you can see a wide variety of examples are possible for anomaly detection.
Reading articles about anomaly detection has been rewarding this week. It is interesting to see how people are building machine learning models, supervising machine learning models, and just trying out new techniques to see what will happen. It's much easier to mock up and work with the security side of data. You can create synthetic data streams and work with models in ways that are not possible for the medical space.[2] I ended up reading that the FDA administration proposed a regulatory framework.[3] That made me start to wonder if that FDA proposed framework had been approved. It seems like they are putting a lot of consideration into that April 2, 2019, discussion paper in the form of premarket reviews.[4] I’m still trying to dig in a little bit more about the approval process to really understand what models are being approved. Overall the consideration and approval of models in the security space are used and deployed without restriction. That is very different from how models are used in the medical research space.
Spending some time thinking and looking at what publicly available medical research datasets exist could be a topic of its own. I’m going to go ahead and add that to the list for future discussion.
Links and thoughts:
This was a very interesting video to watch… “110-year-old Photo Restoration Using A.I.”
“[ML News] OpenAI removes GPT-3 waitlist | GauGAN2 is amazing | NYC regulates AI hiring tools”
“13.2 Filter Methods for Feature Selection -- Variance Threshold (L13: Feature Selection)”
“YouTube Rewind is Back and You CAN'T Dislike It! - WAN Show December 10, 2021”
“AI Show Live - Episode 43 - Responsible AI Dashboard and more!”
Top 5 Tweets of the week:





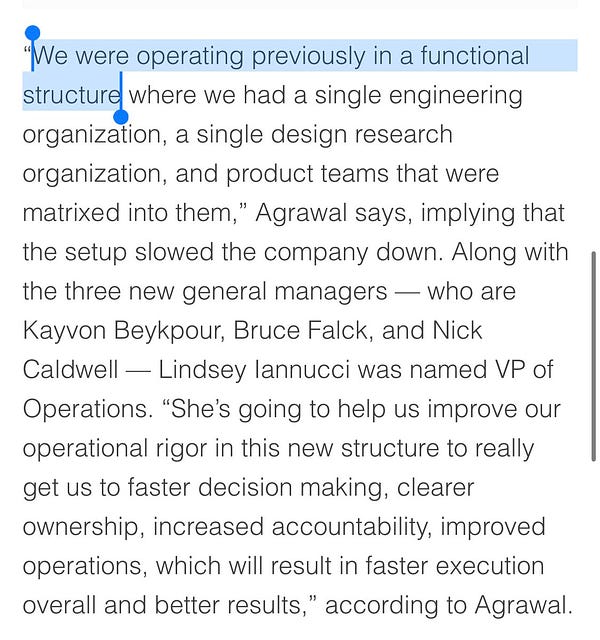
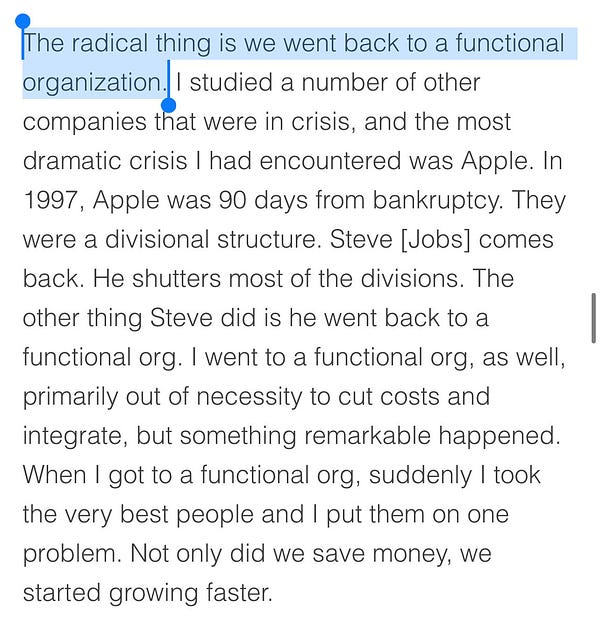

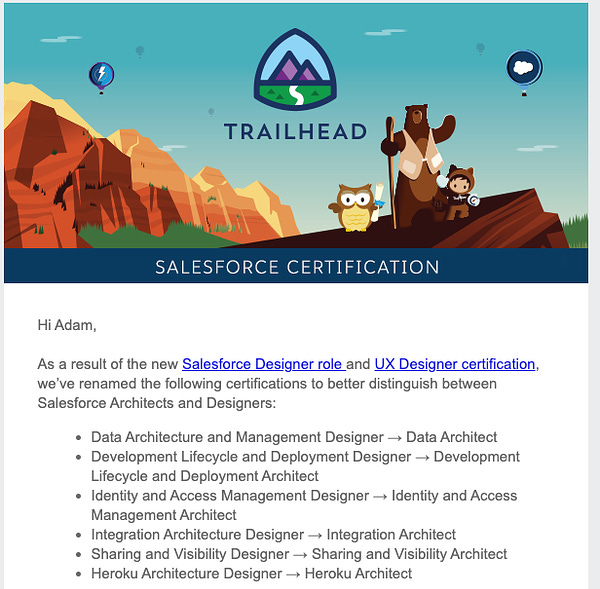
Footnotes:
[1] http://ftp.cerias.purdue.edu/pub/papers/terran-lane/brodley-lane-nissc97_paper.pdf
[2] https://www.nature.com/articles/s41551-021-00751-8
What’s next for The Lindahl Letter?
Week 48: Machine learning applications revisited
Week 49: Machine learning assets
Week 50: Is machine learning the new oil?
Week 51: What is scientific machine learning?
Week 52: That one with a machine learning post
I’ll try to keep the what’s next list forward looking with at least five weeks of posts in planning or review. If you enjoyed reading this content, then please take a moment and share it with a friend.