AIOps/MLOps: Consumption of AI Services vs. operations
A new topic just got added to the end of the running future publication list kept at the end of this document. The United Kingdom just released a National Artificial Intelligence strategy geared at, “The National AI Strategy builds on the UK’s strengths but also represents the start of a step-change for AI in the UK, recognizing the power of AI to increase resilience, productivity, growth and innovation across the private and public sectors.”[1] I’m already starting to work on the “Week 56: Comparative analysis of national AI strategies” Substack post which will include some analysis of the United States, United Kingdom, and China’s AI strategies. So you have that gem of intellectual audacity to look forward to probably next year based on the publishing schedule. This is a topic I’m considering writing a paper on at some point. I believe it is something worth much deeper consideration.
All right back to the topic at hand related to artificial intelligence operations (AIOps) and machine learning operations (MLOps). The other day somebody tried to persuade me to consider ModelOps as a major part of the equation. A number of my talks that you have seen are studies into the world of open source MLOps technology and companies. That research trajectory has never really veered over into the world of ModelOps or the rabbit hole of companies that relate to graphs. Now from time to time the graph based things are really interesting and people sell them with a very passionate series of pitches. This topic however was geared toward digging into the difference between AI services and operations at the point of consumption. A quick search out into Google Scholar for “AIOps” will bring you about 657 results.[2] Some of those results are a bit interesting, but the most relevant result is very short and has only been cited 59 times.[3] That work seems to reference out AIOps and be focused on a website from Moogsoft which is an interesting read.[4] My work really focuses on open source software related to MLOps which is a very different set of space compared to the people who talk about AIOps. For the most part MLOps is directed at how you build out the tooling and workflow to operate machine learning models in production.
Links and thoughts:
Yannic this week had some fun, “[ML News] New ImageNet SOTA | Uber's H3 hexagonal coordinate system | New text-image-pair dataset”
Do you watch the weely Microsoft Developer AI Show each week? This week was called, “AI Show Live - Episode 32 - 2021 OpenCV AI Competition Winners - Cortic Tigers”
This week Linus and Luke were super excited about technology, “Valve Did the IMPOSSIBLE... Anti-Cheat on Linux - WAN Show September 24, 2021”
Top 5 Tweets of the week:

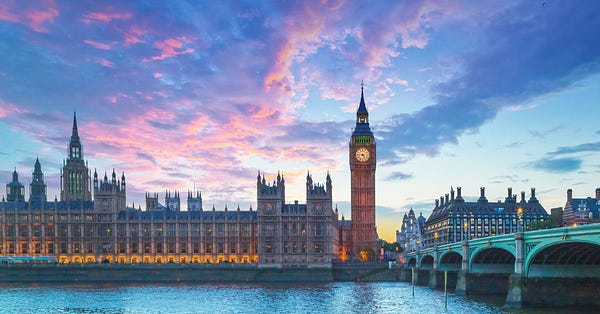

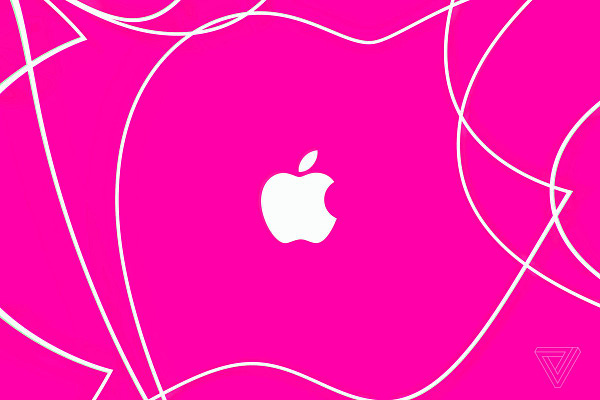

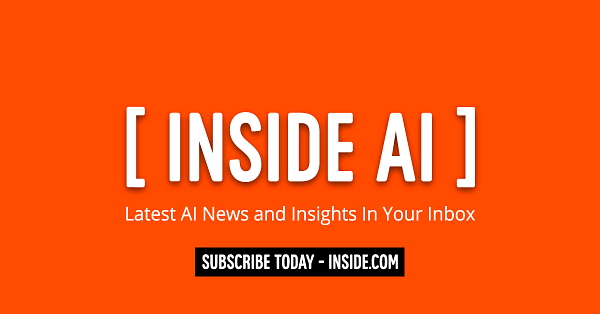

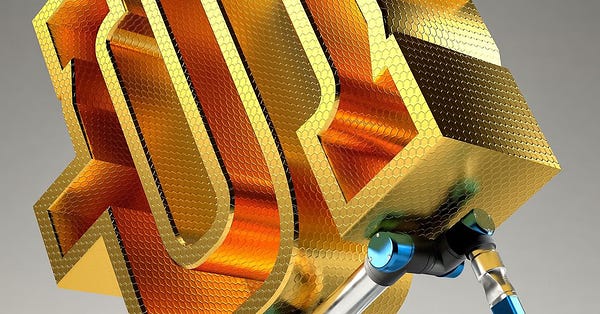

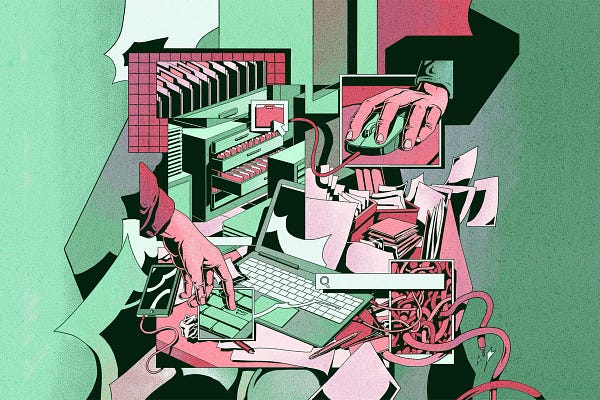
Footnotes:
[1] https://www.gov.uk/government/publications/national-ai-strategy
[2] https://scholar.google.com/scholar?hl=en&as_sdt=0%2C6&q=AIOPs&btnG=
[3] https://ieeexplore.ieee.org/abstract/document/8802836
[4] https://www.moogsoft.com/resources/aiops/guide/everything-aiops/
What’s next for The Lindahl Letter?
Week 37: Reverse engineering GPT-2 or GPT-3
Week 38: Do most ML projects fail?
Week 39: Machine learning security
Week 40: Applied machine learning skills
Week 41: Machine learning and the metaverse
I’ll try to keep the what’s next list forward looking with at least five weeks of posts in planning or review. If you enjoyed reading this content, then please take a moment and share it with a friend.